last updated: December 15, 3p
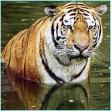
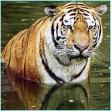
COSC 6397--- Data Mining Fall 2005
(Dr. Eick )
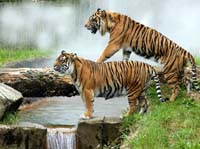
Teaching Assistant: Rachsuda Jiamthapthaksin
www: Rachsuda's Website
e-mail: rachsuda@cs.uh.edu
office hours: TU 10-11a TH 11a-noon
Goals of the Data Mining Course
Data mining centers on finding novel, interesting, and potentially useful patterns in data.
It aims at transforming a large amount of data into a well of knowledge. Data mining
has become a very important field in industry as well as academia. For example, 630 papers were submitted
for the 2005 IEEE International Conference
on Data Mining (ICDM). Data mining tools and
suites (for example, see KDnuggets' DM Software
Survey) are used a lot in industry and
in reseach projects.
UH's Data Mining and Machine Learning Group
(UH-DMML) conducts research in some of the areas that are covered by this course.
The course discusses the most important
data mining techniques and provides background knowledge on how to approach a data mining task. Moreover, the
application of data mining techniques will be studied in homework assignements and a course project.
Comments concerning this website
If you have any comments
concerning this website, send e-mail
to: ceick@aol.com
Basic Course Information
Instructor: Dr.
Christoph F. Eick
office hours (589 PGH): TU 2:30-3:30p TH 11:30a-12:30p
TA: ?
office: ??? PGH
e-mail: ???@cs.uh.edu
officehours: ??p
class meets: TU/TH 1-2:30p in 232 PGH
cancelled classes: Tu., Nov. 29. 2005
makeup classes: Friday, Nov. 11, 2-5p (double makeup class for Rita and Nov. 29, 2005)
class room: 232 PGH
Course Materials
Required Text:
- Jiawei Han and Micheline Kamber, Data Mining: Concepts and
Techniques
- Morgan Kaufman Publishers, 2001, ISBN 1-55860-489-8
- Link to Data Mining Book Home
Page
Recommended Text
- P.-N. Tang, M. Steinback, and V. KumarIntroduction to Data Mining,
- Addison Wesley,
- Link to
Book HomePage
News COSC 6367 (Data Mining) Fall 2005
- The grades should be available Thursday night; check Rachsuda webpage after 6p. Solutions
to some homework problems can also be found on her website.
- The final exam will not be returned to students; however, you can look at your final exam:
Tu., Jan. 4, 11a-noon; Th., Jan. 20, 4:30-5:30p; Tu., Jan. 25, 5-6p.
- Solution Sketches for Homework1, for a
few problems of Homework2, and for Exam/Quiz1 are available now!
- Due to the fact that the course is offered the first time, the teaching plan is
preliminary and subject to change.
- Approx. 80% of the teaching material covered in the class orginates from the Han and Tan introductions to data
mining.
Prerequisites
The course is mostly self-contained. However, students talking the course should have
sound software development skills. Lacking these skills likely will ask for trouble when performing
the course projects.
2005 Homeworks, Projects and Assignments
Homework1 (due on Th., Sept. 29 in class)
Homework2 (due on Mo., October 10, 10a --- electronic submission)
Homework3 (due on Tu., Nov. 8, in class)
Homework4 (due on Sa., Dec. 3, 11p, electronic submission)
Course Elements
1 quiz(first week of October), 1 midterm (Tu., November 15, 2005), and 1 final exam
1 paper review
4 assignments/homeworks (contain medium-size KDD projects, short review problems, and tasks some of which
might involve some programming or using a KDD-tool; other problems are intended as a prepartion for the exams
of the course)
Due Dates and Exam Dates 2005
Homework1: Th., Sept. 27, 2005, in class
Homework2: Mo., October 10, 10a (electronic submission)
Exam1: Th., October 13
Homework3: Tu., November 8, in class
Exam2: Th., November 10, 2005
Homework4: Sa., Dec. 3, 2005
Final Exam: ...
Class Transparencies
I Introduction to Data Mining (Part1,
Part2 (covers Chapter1 of the Han book))
II Exploratory Data Analysis (the transparencies are "self-explanatory")
III Data Preprocessing (covers Han Chapter3; finalized set of
transparencies to be used in the second week of the semester)
IV Concept Description (Han Chapter5; transparency 40 and higher
will not be discussed in the lecture)
V Introduction to Classification: Basic Concepts and
Decision Trees (last transparency modified and 4 transparencies added on Sept. 28, 2005)
VI More on Classification: Instance-based Learning and Support Vector Machines
(NN-Classifiers and Support Vector Machines (updated on Sept. 28, 2005),
Editing and Condensing Techniques for NN-Classifiers)
VII Introduction to Similarity Assessment and Clustering
VIII More on Clustering: Grid-based, Hierarchical and Density-based Clustering
(more on AGNES and DBSCAN),
Critical Issues with Respect to Clustering",
Supervised Clustering.
IX Brief Introduction to Data Cubes (Han Chapter 2 centering on cubes)
X Association Rules
XI Paper Walk Throughs
XII Mining Complex Types of Data Part1, Part2 (covers Han Chapter 9
in part)
XIII Spatial Data Mining (Spatial Databases,
Spatial Data Mining (very long),
Spatial Data Mining Transparencies covered in class)
XIV Final Words
Remark: Topics with '*' might not be covered due to a lack of time.
2005 Paper Walk Throughs
The paper walk through will take place during the makeup class on Fr., November 11, 2005 from 2-5p.
The following 2 papers will be discussed: Original DBSCAN Paper, Adding Data
Mining Capabilities to Data Cubes Paper. Everybody should read the two papers carefully. Students are assigned to
different Sections of the Paper and should be prepared to summarize the contents of their assigned section and are in charge
of directing the discussion of the paper. Students are also assigned as backups and should be
prepared to help the student in charge of discussing the paper, and are expected to
actively particiapte in the discussion of this section. In the case, that you do not understand something,
write down what you do not understand in form of a question.
Student Assignments for the DBSCAN-Paper:
Section 1+2: Wu, Ma
Section 3: Jefferson, Thomas
Section 4, 4.1: Wadwani, Patel
Section 4.2: Ma, Wu
Section 5: Thomas, Jefferson
Section 6: Patel, Wadwami
Student Assignments for the Sarawawi Paper:
Section 1: Arigai, Belokrylov
Section 2: Alkan, Vaezian
Sections 3.1, 3.2, 3.3: Belokrylov, Arigai
Section 3.4, 3.5, 3.6: Saveran, Tabbaa
Section 4.1: Vaezian, Alkan
Section 4.2, 5: Tabbaa, Saverna
Grading
Each student has to
have a weighted average of 74.0 or higher in the
exams of the course in order to receive a grade of "B-" or better
for the course.
Students will be responsible for material covered in the
lectures and assigned in the readings. All homeworks and
project reports are due at the date specified.
No late submissions
will be accepted after
the due date. This policy will be strictly enforced.
Translation number to letter grades:
A:100-90 A-:90-86 B+:86-82 B:82-77 B-:77-74 C+:74-70
C: 70-66 C-:66-62 D+:62-58 D:58-54 D-:54-50 F: 50-0
Only machine written solutions to homeworks and assignments
are accepted (the only exception to this point are figures and complex formulas) in the assignments.
Be aware of the fact that our
only source of information is what you have turned in. If we are not capable to understand your
solution, you will receive a low score.
Moreover, students should not throw away returned assignments or tests.
Students may discuss course material and homeworks, but must take special
care to discern the difference between collaborating in order to increase
understanding of course materials and collaborating on the homework /
course project
itself. We encourage students to help each other understand course
material to clarify the meaning of homework problems or to discuss
problem-solving strategies, but it is not permissible for one
student to help or be helped by another student in working through
homework problems and in the course project. If, in discussing course materials and problems,
students believe that their like-mindedness from such discussions could be
construed as collaboration on their assignments, students must cite each
other, briefly explaining the extent of their collaboration. Any
assistance that is not given proper citation may be considered a violation
of the Honor Code, and might result in obtaining a grade of F
in the course, and in further prosecution.
Course Exams
Quiz
Midterm
Final Exam
Data Mining Links
KDnuggets
KDD 2005 Conference
IEEE International Conference
on Data Mining (ICDM) Website
PKDD 2005 (European KDD Conference)
UIUC Data Mining Group
Microsoft DMX Group
Penn Data Mining Group
UMN Spatial Database and Spatial Data Mining Group
Vrije Universiteit Amsterdam Data Mining Group
Data Mining and Machine Learning Group University
of Helsinki
Data Mining at Massey University
UH's Data Mining and Machine Learning Group (UH-DMML)